Document (5) - Extract from Volkswagen Transporter/Caravelle sales brochure from 1980 from UK
To upload the documents, we need to first create the necessary database to hold the documents, user and warehouse to be used later with the agent gateway configuration.
-- ======================================== -- Set active role with full admin privileges -- ======================================== USE ROLE ACCOUNTADMIN;
-- ======================================== -- Create database and schema for storing agent data -- ======================================== CREATE DATABASE IF NOT EXISTS agent_gateway; USE DATABASE agent_gateway;
CREATE SCHEMA IF NOT EXISTS ag_schema;
-- ======================================== -- Create a virtual warehouse to process queries and tasks -- ======================================== CREATE OR REPLACE WAREHOUSE agent_wh; USE WAREHOUSE agent_wh;
-- ======================================== -- Create custom Cortex role and link it to Snowflake Cortex privileges -- ======================================== CREATE OR REPLACE ROLE cortex_user_role; GRANT DATABASE ROLE SNOWFLAKE.CORTEX_USER TO ROLE cortex_user_role;
-- ======================================== -- Create users and assign roles -- ======================================== CREATE OR REPLACE USER agent_user; GRANT ROLE cortex_user_role TO USER agent_user;
-- Assign to your user (replace with real username if needed) GRANT ROLE cortex_user_role TO USER mheino;
-- ======================================== -- Grant necessary warehouse permissions -- ======================================== GRANT USAGE ON WAREHOUSE agent_wh TO ROLE cortex_user_role; GRANT OPERATE ON WAREHOUSE agent_wh TO ROLE cortex_user_role;
-- ======================================== -- Grant full access to the database and schema -- ======================================== GRANT ALL ON DATABASE agent_gateway TO ROLE cortex_user_role; GRANT ALL ON SCHEMA ag_schema TO ROLE cortex_user_role;
-- ======================================== -- Set password for agent_user (not secure for prod environments) -- ======================================== ALTER USER agent_user SET PASSWORD = 'agent_user' MUST_CHANGE_PASSWORD = FALSE;
-- ======================================== -- Switch to cortex role to begin resource creation -- ======================================== USE ROLE cortex_user_role;
|
Once we have our database setup, we can create a stage to store the documents. Uploading files is easy through the UI. In our use case, the maximum file size of 250 MB required for compressing a few original files. Splitting files would also work.
-- ======================================== -- Create external stages for PDF documents and repair manuals -- These are used as file storage sources -- ========================================
-- Stage for storing VW documents create or replace stage agent_gateway.ag_schema.vw directory = (enable = true) encryption = (type = 'SNOWFLAKE_SSE');
-- Stage for storing VW repair manuals create or replace stage agent_gateway.ag_schema.repair_manual directory = (enable = true) encryption = (type = 'SNOWFLAKE_SSE');
-- Stage for storing Cortex Analyst semantic model CREATE STAGE public DIRECTORY = (ENABLE = TRUE); GRANT READ ON STAGE public TO ROLE PUBLIC;
|
Cortex Parse Document modes (OCR and Layout)
Before we can start extracting data from our documents, we need to understand what modes Parse Document offers. You get information out of PDF files in two different ways: OCR (which is the default) and LAYOUT.
OCR Mode (Default):
This mode is the default for pulling out text from documents that mostly just contain words. It’s the best choice when you just want to grab the content quickly and don’t need to worry about how the page is structured.
LAYOUT Mode (Optional):
This mode not only pulls out the text but also keeps track of how things are arranged on the page — like tables or sections. It’s useful when the structure of the document matters, such as when building a smarter search system or helping AI tools better understand the content. By keeping the layout in mind, it’s easier to focus on specific parts of the document and get more accurate results.
In our use case, the default OCR mode is better, because our documents are not in any case the best suitable documents for Parse Document.
Cortex Parse Document limitations and considerations
Although PARSE_DOCUMENT function is a powerful tool, there are a few limitations to keep in mind:
- File Size & Page Limits: It supports PDF files up to 100MB and up to 300 pages.
- Language Support: It works best with English. Support for other languages like French and Portuguese is still in preview.
- Digital PDF Optimization: It’s designed for PDFs created digitally. Supports several typical image-formats (PDF, PPTX, DOCX, JPEG, JPG, PNG, TIFF, TIF). Scanned documents might not work as well.
- Stage Encryption: The stage you use must have server-side encryption enabled.
- Network Policy Compatibility: Custom network policies aren’t supported at the moment.
Our documents, as shown above, are not ideal for a Cortex Parse document, but the documents also reflect reality. A massive amount of documents on the internet are not digital PDFs and thus the sizes of the documents are bigger than 100MB or 100 pages, when dealing with maintenance manuals with large images for example.
Uploading documents through PARSE_DOCUMENT happens easily (if we choose document one-by-one).
-- Create table to store parsed documents create or replace table parsed_documents ( file_name string, content string );
-- Query documents within external stage select distinct metadata$filename as file_name from @agent_gateway.ag_schema.vw;
-- Parse documents and insert them into parsed_documents insert into parsed_documents select file_name, parse_result:content::string as content from ( select 'transporter-with-wbx-vag-self-study-programme.pdf' as file_name, snowflake.cortex.parse_document( @agent_gateway.ag_schema.vw, 'transporter-with-wbx-vag-self-study-programme.pdf', {'mode': 'OCR'} ) as parse_result );
|
The results are good for non-digital documents, as seen in the example. Parse document was able to extract text from different colour backgrounds and from text that use type-writer fonts, not traditionally used in digital PDFs.
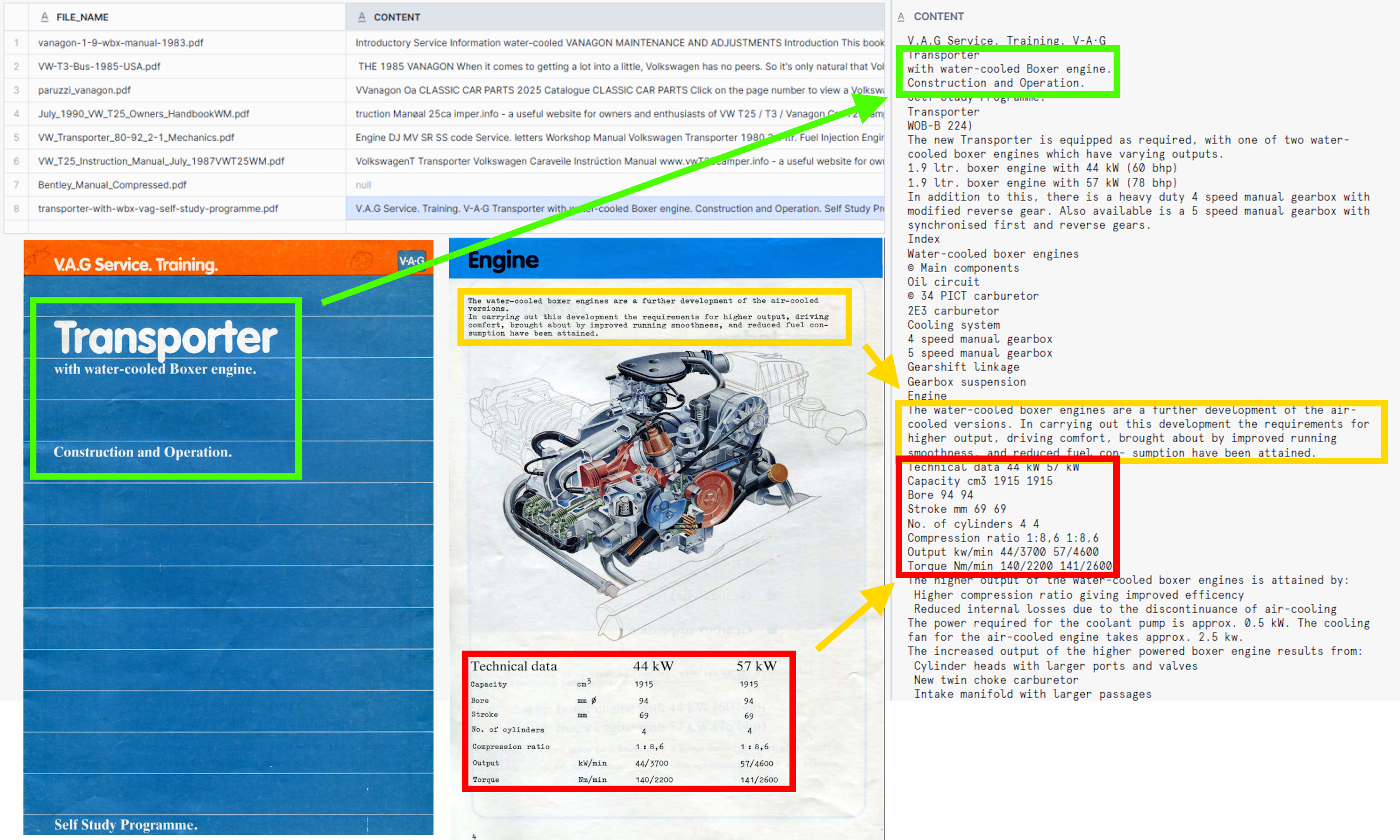
Even though the results are good, one can see that the output is not usable in my use case. Cortex Parse document does not support chucking parsed content. The whole text is parsed into one column. This means that the Cortex Parse document is a good option for extracting simple PDFs, such as invoices, BOLs and receipts - documents where the whole content is within a few pages.
This means that I need to revisit my original workflow and use PyPDF if I want to have good results later with Cortex Search. Cortex Search has a recommendation of “splitting the text in your search column into chunks of no more than 512 tokens (about 385 English words).” because “a smaller chunk size typically results in higher retrieval and downstream LLM response quality.”
Before jumping into PyPDF, I want to say that Cortex family has a function called SPLIT_TEXT_RECURSIVE_CHARACTER which can do almost all the stuff that I’m going to implement in my Python function, such as chunk size, overlap and separators functionality.
This means that I could try to build something like this, but I would still have issues with page numbers.
SELECT SNOWFLAKE.CORTEX.SPLIT_TEXT_RECURSIVE_CHARACTER( SNOWFLAKE.CORTEX.PARSE_DOCUMENT( '@your_stage', 'your_document.pdf', {'mode': 'OCR'} ):content, 'none', 1000, 100 ) AS text_chunks; |
Separators could be used to identify page numbers – which is usually, because I want to open the original document with associated pictures. Separators rely on chucked text containing page number to use a separator, whereas PyPDF uses internal reader.pages[i] to expose internal PDF page tree.
PyPDF (Snowpark Function) initialize & configuration
Using PyPDF is really easy with Snowpark and we do have several examples which I can copy to use. For this blog, I modified the PyPDF code from an older QS of Cortex Search. Using PyPDF is easy, I’ve already used to parse VW1300 documents in my Youtube -video (I do have a Beetle as well).
This Snowpark code creates a Python function that reads PDF -document, adds page number and creates chunks per page with a chuck overlap between previous and next page to increase search quality.
-- ======================================== -- Create Python UDF to chunk PDF documents by page with overlap -- This function adds overlap between adjacent pages for context -- ======================================== CREATE OR REPLACE FUNCTION agent_gateway.ag_schema.pdf_text_chunker(file_url STRING) RETURNS TABLE (chunk VARCHAR, page_number INT) LANGUAGE PYTHON RUNTIME_VERSION = '3.9' HANDLER = 'pdf_text_chunker' PACKAGES = ('snowflake-snowpark-python', 'PyPDF2', 'langchain') AS $$ from snowflake.snowpark.types import StringType, StructField, StructType from langchain.text_splitter import RecursiveCharacterTextSplitter from snowflake.snowpark.files import SnowflakeFile import PyPDF2 import io import logging import pandas as pd
class pdf_text_chunker:
def read_pdf(self, file_url: str): logger = logging.getLogger("udf_logger") logger.info(f"Opening file {file_url}")
with SnowflakeFile.open(file_url, 'rb') as f: buffer = io.BytesIO(f.readall())
reader = PyPDF2.PdfReader(buffer) page_texts = [] for i, page in enumerate(reader.pages): try: text = page.extract_text().replace('\n', ' ').replace('\0', ' ') page_texts.append((text, i + 1)) # 1-based page indexing except Exception as e: logger.warning(f"Unable to extract from file {file_url}, page {i + 1}: {e}") page_texts.append(("Unable to Extract", i + 1))
return page_texts
def process(self, file_url: str): page_texts = self.read_pdf(file_url) num_pages = len(page_texts)
text_splitter = RecursiveCharacterTextSplitter( chunk_size=2000, chunk_overlap=300, length_function=len )
chunks_with_page_numbers = [] for i, (text, page_num) in enumerate(page_texts): prev_overlap = page_texts[i - 1][0][-300:] if i > 0 else '' next_overlap = page_texts[i + 1][0][:300] if i < num_pages - 1 else '' combined_text = f"{prev_overlap} {text} {next_overlap}".strip() chunks = text_splitter.split_text(combined_text) for chunk in chunks: chunks_with_page_numbers.append((chunk, page_num))
df = pd.DataFrame(chunks_with_page_numbers, columns=['chunk', 'page_number']) yield from df.itertuples(index=False, name=None) $$; |
This function is used in the following manner.
Reading individual document:
-- ======================================== -- Test the PDF chunker using a sample PDF from VW stage -- ======================================== SELECT * FROM TABLE(agent_gateway.ag_schema.pdf_text_chunker( BUILD_SCOPED_FILE_URL(@agent_gateway.ag_schema.vw, 'transporter-with-wbx-vag-self-study-programme.pdf') ));
|
Which results in the following output.
Reading several documents from the external stage and creating a table to store output with necessary metadata of the parsed document is done with following code:
-- ======================================== -- Chunk all files in the VW document stage and store in a table -- ======================================== CREATE OR REPLACE TABLE agent_gateway.ag_schema.man_chunks_vw_documents AS SELECT relative_path, build_scoped_file_url(@agent_gateway.ag_schema.vw, relative_path) AS file_url, CONCAT(relative_path, ': ', func.chunk) AS chunk, func.page_number, 'English' AS language FROM directory(@agent_gateway.ag_schema.vw), TABLE(agent_gateway.ag_schema.pdf_text_chunker( build_scoped_file_url(@agent_gateway.ag_schema.vw, relative_path) )) AS func; |
This results into following table, which contains
- Original PDF name (relative_path)
- URL to PDF location with stage, can be used to download original document (FILE_URL)
- Chuck with name of the original pdf name and overlap from previous and next chunk
- Page number of the original document
- Language (hardcoded, but acts as placeholder)
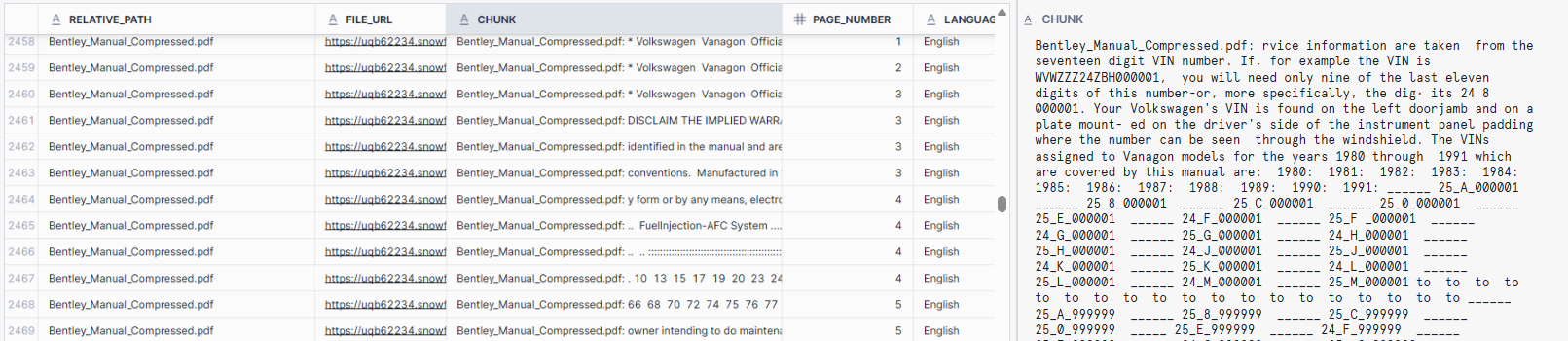
Now the data looks good for Cortex Search usage, so we can continue with Cortex Search initialization.
Cortex Search initialize & configuration
What is Cortex Search? Cortex Search is a search function that enables high-quality “fuzzy” search over your data. Under the hood, Cortex Search takes a hybrid approach to retrieving and ranking documents. Each search query utilizes:
- Vector search for retrieving semantically similar documents.
- Keyword search for retrieving lexically similar documents.
- Semantic reranking for reranking the most relevant documents in the result set.
Initializing Cortex Search happens by creating a search service from your text chunks. As noted above, Cortex Search works best with small chunks of data, recommending 512 tokens (about 385 English words). When the chuck of data is within 512 tokens, it uses all approaches of document retrieval. If the chuck (entire body of the text) is longer than 512 tokens, Cortex uses keyword search for the whole body of text, but uses only 512 tokens for semantic retrieval.
Cortex Search has few parameters that you can tweak.
- Query column (in our example chunk contains the body of text to search)
- Attributes as filter (in our example relative_path, which is the original document name)
- Warehouse, which is used in materializing the results
- Target lag, which dictates how often Search service will check updates on the base table (for new documents in this pattern)
- Embedding model (which differ from credit cost as they differ in parameter size)
- Finally the select query defines columns that are also indexed and returned together with query (we will use relative_path, file_url, chunk and page_number)
-- ======================================== -- Create Cortex Search Service for VW documents -- Enables semantic document search with hourly refresh -- ======================================== CREATE OR REPLACE CORTEX SEARCH SERVICE agent_gateway.ag_schema.vw_documents ON chunk ATTRIBUTES relative_path WAREHOUSE = agent_wh TARGET_LAG = '1 hour' EMBEDDING_MODEL = 'snowflake-arctic-embed-m-v1.5' AS ( SELECT relative_path, file_url, chunk, page_number FROM agent_gateway.ag_schema.man_chunks_vw_documents ); |
Known limitations are that the result query must not exceed 100M of rows as the results result in query failure.
Testing Cortex Search
-- ======================================== -- Sample query to test VW document search service -- ======================================== SELECT PARSE_JSON( SNOWFLAKE.CORTEX.SEARCH_PREVIEW( 'agent_gateway.ag_schema.vw_documents', '{ "query": "what diesel options do I have", "columns":[ "relative_path", "file_url", "chunk", "page_number" ], "limit":1 }' ) )['results'] AS results; |
Which gives us really promising answers as seen below
Cortex Analyst initialize & configuration
What is a Cortex Analyst? Cortex Analyst is a fully managed service within Snowflake Cortex that enables users to interact with structured data using natural language. It translates user queries into SQL, providing accurate answers without the need for SQL expertise. You can think of Cortex Analyst as a sidekick for Cortex Search. Whereas Cortex Search worked for unstructured data, Analyst does the same for structured data.
To use Cortex Analyst, we need to initialize it with structured data. For this purpose I have included a few tables that contain master data information about Volkswagen Transporter T2.5/T3 VINs and chassis codes. With this information we can identify any Transporter just by their VIN number.
--- Cortex Analyst Setup ==================================================================== -- Table Creation: Type25ChassisCodes -- Purpose: Stores chassis code information for Type 25 vehicles, -- including the production month, year, and chassis number. -- ==================================================================== CREATE OR REPLACE TABLE AGENT_GATEWAY.AG_SCHEMA.Type25ChassisCodes ( Month VARCHAR(10), -- Production month (e.g., 'May', 'Aug'). Year INT, -- Production year (e.g., 1980, 1981). ChassisNumber VARCHAR(15) -- Unique chassis identifier. );
-- Insert records into the Type25ChassisCodes table. -- Each entry corresponds to a specific production period and chassis number. INSERT INTO AGENT_GATEWAY.AG_SCHEMA.Type25ChassisCodes (Month, Year, ChassisNumber) VALUES ('May', 1980, '24-A-00000001'); INSERT INTO AGENT_GATEWAY.AG_SCHEMA.Type25ChassisCodes (Month, Year, ChassisNumber) VALUES ('Aug', 1980, '24-A-0013069'); INSERT INTO AGENT_GATEWAY.AG_SCHEMA.Type25ChassisCodes (Month, Year, ChassisNumber) VALUES ('Jan', 1980, '25-A-0000410'); INSERT INTO AGENT_GATEWAY.AG_SCHEMA.Type25ChassisCodes (Month, Year, ChassisNumber) VALUES ('Jul', 1980, '24-A-0150805'); INSERT INTO AGENT_GATEWAY.AG_SCHEMA.Type25ChassisCodes (Month, Year, ChassisNumber) VALUES ('Aug', 1981, '24-B-0000001'); INSERT INTO AGENT_GATEWAY.AG_SCHEMA.Type25ChassisCodes (Month, Year, ChassisNumber) VALUES ('Jan', 1981, '24-B-095074'); INSERT INTO AGENT_GATEWAY.AG_SCHEMA.Type25ChassisCodes (Month, Year, ChassisNumber) VALUES ('Jun', 1981, '24-B-175000'); INSERT INTO AGENT_GATEWAY.AG_SCHEMA.Type25ChassisCodes (Month, Year, ChassisNumber) VALUES ('Jul', 1982, '24-C-0000001'); INSERT INTO AGENT_GATEWAY.AG_SCHEMA.Type25ChassisCodes (Month, Year, ChassisNumber) VALUES ('Jan', 1982, '24-C-089151'); INSERT INTO AGENT_GATEWAY.AG_SCHEMA.Type25ChassisCodes (Month, Year, ChassisNumber) VALUES ('Jul', 1982, '24-C-175000'); INSERT INTO AGENT_GATEWAY.AG_SCHEMA.Type25ChassisCodes (Month, Year, ChassisNumber) VALUES ('Aug', 1983, '24-D-0000001'); INSERT INTO AGENT_GATEWAY.AG_SCHEMA.Type25ChassisCodes (Month, Year, ChassisNumber) VALUES ('Jan', 1983, '24-D-062766'); INSERT INTO AGENT_GATEWAY.AG_SCHEMA.Type25ChassisCodes (Month, Year, ChassisNumber) VALUES ('Jul', 1983, '24-D-175000'); INSERT INTO AGENT_GATEWAY.AG_SCHEMA.Type25ChassisCodes (Month, Year, ChassisNumber) VALUES ('Aug', 1984, '24-E-0000001'); INSERT INTO AGENT_GATEWAY.AG_SCHEMA.Type25ChassisCodes (Month, Year, ChassisNumber) VALUES ('Jan', 1984, '24-E-081562'); INSERT INTO AGENT_GATEWAY.AG_SCHEMA.Type25ChassisCodes (Month, Year, ChassisNumber) VALUES ('Jul', 1984, '24-E-175000'); INSERT INTO AGENT_GATEWAY.AG_SCHEMA.Type25ChassisCodes (Month, Year, ChassisNumber) VALUES ('Aug', 1985, '24-F-0000001'); INSERT INTO AGENT_GATEWAY.AG_SCHEMA.Type25ChassisCodes (Month, Year, ChassisNumber) VALUES ('Jan', 1985, '24-F-073793'); INSERT INTO AGENT_GATEWAY.AG_SCHEMA.Type25ChassisCodes (Month, Year, ChassisNumber) VALUES ('Jul', 1985, '24-F-175000'); INSERT INTO AGENT_GATEWAY.AG_SCHEMA.Type25ChassisCodes (Month, Year, ChassisNumber) VALUES ('Aug', 1986, '24-G-0000001'); INSERT INTO AGENT_GATEWAY.AG_SCHEMA.Type25ChassisCodes (Month, Year, ChassisNumber) VALUES ('Jan', 1986, '24-G-068279'); INSERT INTO AGENT_GATEWAY.AG_SCHEMA.Type25ChassisCodes (Month, Year, ChassisNumber) VALUES ('Jul', 1986, '24-G-175000'); INSERT INTO AGENT_GATEWAY.AG_SCHEMA.Type25ChassisCodes (Month, Year, ChassisNumber) VALUES ('Aug', 1987, '24-H-0000001'); INSERT INTO AGENT_GATEWAY.AG_SCHEMA.Type25ChassisCodes (Month, Year, ChassisNumber) VALUES ('Jan', 1987, '24-H-072878'); INSERT INTO AGENT_GATEWAY.AG_SCHEMA.Type25ChassisCodes (Month, Year, ChassisNumber) VALUES ('Jul', 1987, '24-H-175000'); INSERT INTO AGENT_GATEWAY.AG_SCHEMA.Type25ChassisCodes (Month, Year, ChassisNumber) VALUES ('Aug', 1988, '24-J-0000001'); INSERT INTO AGENT_GATEWAY.AG_SCHEMA.Type25ChassisCodes (Month, Year, ChassisNumber) VALUES ('Jan', 1988, '24-J-060498'); INSERT INTO AGENT_GATEWAY.AG_SCHEMA.Type25ChassisCodes (Month, Year, ChassisNumber) VALUES ('Jul', 1988, '24-J-120000'); INSERT INTO AGENT_GATEWAY.AG_SCHEMA.Type25ChassisCodes (Month, Year, ChassisNumber) VALUES ('Aug', 1989, '24-K-0000001'); INSERT INTO AGENT_GATEWAY.AG_SCHEMA.Type25ChassisCodes (Month, Year, ChassisNumber) VALUES ('Jan', 1989, '24-K-077876'); INSERT INTO AGENT_GATEWAY.AG_SCHEMA.Type25ChassisCodes (Month, Year, ChassisNumber) VALUES ('Jul', 1989, '24-K-175000'); INSERT INTO AGENT_GATEWAY.AG_SCHEMA.Type25ChassisCodes (Month, Year, ChassisNumber) VALUES ('Aug', 1990, '24-L-0000001'); INSERT INTO AGENT_GATEWAY.AG_SCHEMA.Type25ChassisCodes (Month, Year, ChassisNumber) VALUES ('Jan', 1990, '24-L-056781'); INSERT INTO AGENT_GATEWAY.AG_SCHEMA.Type25ChassisCodes (Month, Year, ChassisNumber) VALUES ('Jul', 1990, '24-L-175000'); INSERT INTO AGENT_GATEWAY.AG_SCHEMA.Type25ChassisCodes (Month, Year, ChassisNumber) VALUES ('Aug', 1991, '24-M-0000001'); INSERT INTO AGENT_GATEWAY.AG_SCHEMA.Type25ChassisCodes (Month, Year, ChassisNumber) VALUES ('Jan', 1991, '24-M-010527'); INSERT INTO AGENT_GATEWAY.AG_SCHEMA.Type25ChassisCodes (Month, Year, ChassisNumber) VALUES ('Jul', 1991, '24-M-020000'); INSERT INTO AGENT_GATEWAY.AG_SCHEMA.Type25ChassisCodes (Month, Year, ChassisNumber) VALUES ('Aug', 1992, '24-N-002182'); INSERT INTO AGENT_GATEWAY.AG_SCHEMA.Type25ChassisCodes (Month, Year, ChassisNumber) VALUES ('Jan', 1992, '24-N-002183'); INSERT INTO AGENT_GATEWAY.AG_SCHEMA.Type25ChassisCodes (Month, Year, ChassisNumber) VALUES ('Jul', 1992, '24-N-015000');
-- ==================================================================== -- Table Creation: CountryOfManufacture -- Purpose: Maps country codes to their respective country names. -- ==================================================================== CREATE OR REPLACE TABLE AGENT_GATEWAY.AG_SCHEMA.CountryOfManufacture ( Code CHAR(1) PRIMARY KEY, -- Single-character country code (e.g., 'W'). Country VARCHAR(50) -- Full country name (e.g., 'Germany'). );
-- Insert the country code for Germany. INSERT INTO AGENT_GATEWAY.AG_SCHEMA.CountryOfManufacture (Code, Country) VALUES ('W', 'Germany');
-- ==================================================================== -- Table Creation: Manufacturer -- Purpose: Maps manufacturer codes to their respective manufacturer names. -- ==================================================================== CREATE OR REPLACE TABLE AGENT_GATEWAY.AG_SCHEMA.Manufacturer ( Code CHAR(1) PRIMARY KEY, -- Single-character manufacturer code (e.g., 'V'). Name VARCHAR(50) -- Manufacturer name (e.g., 'Volkswagen'). );
-- Insert the manufacturer code for Volkswagen. INSERT INTO AGENT_GATEWAY.AG_SCHEMA.Manufacturer (Code, Name) VALUES ('V', 'Volkswagen');
-- ==================================================================== -- Table Creation: VehicleBodyType -- Purpose: Defines various vehicle body types associated with specific codes. -- ==================================================================== CREATE OR REPLACE TABLE AGENT_GATEWAY.AG_SCHEMA.VehicleBodyType ( Code CHAR(1) PRIMARY KEY, -- Single-character body type code (e.g., '1'). Description VARCHAR(50) -- Description of the body type (e.g., 'Pickup Truck'). );
-- Insert records for different vehicle body types. INSERT INTO AGENT_GATEWAY.AG_SCHEMA.VehicleBodyType (Code, Description) VALUES ('1', 'Pickup Truck'), ('2', 'MPV (Multi-Purpose Vehicle)');
-- ==================================================================== -- Table Creation: VehicleSeries -- Purpose: Defines various vehicle series associated with specific codes. -- ==================================================================== CREATE OR REPLACE TABLE AGENT_GATEWAY.AG_SCHEMA.VehicleSeries ( Code CHAR(1) PRIMARY KEY, -- Single-character series code (e.g., 'U'). Description VARCHAR(50) -- Description of the vehicle series (e.g., '1980-91 Single-Cab Pickup (Pritschewagen)'). );
-- Insert records for different vehicle series. INSERT INTO AGENT_GATEWAY.AG_SCHEMA.VehicleSeries (Code, Description) VALUES ('U', '1980-91 Single-Cab Pickup (Pritschewagen)'), ('V', '1980-91 Double-Cab Pickup (Doppelkabine)'), ('W', '1980-91 Panel Van (no side windows)'), ('X', '1980-91 Kombi'), ('Y', '1980-91 Bus (Vanagon)'), ('Z', '1980-91 Camper');
-- ==================================================================== -- Table Creation: YearOfManufacture -- Purpose: Maps year codes to their respective manufacturing years. -- ==================================================================== CREATE OR REPLACE TABLE AGENT_GATEWAY.AG_SCHEMA.YearOfManufacture ( Code CHAR(1) PRIMARY KEY, -- Single-character year code (e.g., 'B'). Year INT -- Corresponding manufacturing year (e.g., 1981). );
-- Insert records mapping year codes to actual years. INSERT INTO AGENT_GATEWAY.AG_SCHEMA.YearOfManufacture (Code, Year) VALUES ('B', 1981), ('C', 1982), ('D', 1983), ('E', 1984), ('F', 1985), ('G', 1986), ('H', 1987), ('J', 1988), ('K', 1989), ('L', 1990), ('M', 1991);
-- ==================================================================== -- Table Creation: AssemblyPlant -- Purpose: Maps assembly plant codes to their respective locations. -- ==================================================================== CREATE OR REPLACE TABLE AGENT_GATEWAY.AG_SCHEMA.AssemblyPlant ( Code CHAR(1) PRIMARY KEY, -- Single-character assembly plant code (e.g., 'A'). Location VARCHAR(50) -- Location of the assembly plant (e.g., 'Ingolstadt'). );
-- Insert records for different assembly plant locations. INSERT INTO AGENT_GATEWAY.AG_SCHEMA.AssemblyPlant (Code, Location) VALUES ('A', 'Ingolstadt'), ('B', 'Brussels'), ('E', 'Emden'), ('G', 'Graz (Austria for Syncro models)'), ('H', 'Hannover'), ('K', 'Osnabrück'), ('M', 'Mexico'), ('N', 'Neckarsulm'), ('P', 'Brazil'), ('S', 'Stuttgart'), ('W', 'Wolfsburg');
-- ==================================================================== -- Table Creation: VW_Type25_VIN_Master -- Purpose: Central repository for Vehicle Identification Numbers (VINs) -- of Type 25 vehicles, linking to various attribute tables. -- ==================================================================== CREATE OR REPLACE TABLE AGENT_GATEWAY.AG_SCHEMA.VW_Type25_VIN_Master ( VIN VARCHAR(17) PRIMARY KEY, -- 17-character Vehicle Identification Number. CountryOfManufacture CHAR(1), -- Foreign key referencing CountryOfManufacture(Code). Manufacturer CHAR(1), -- Foreign key referencing Manufacturer(Code). VehicleBodyType CHAR(1), -- Foreign key referencing VehicleBodyType(Code). VehicleSeries CHAR(1), -- Foreign key referencing VehicleSeries(Code). YearOfManufacture CHAR(1), -- Foreign key referencing YearOfManufacture(Code). AssemblyPlant CHAR(1), -- Foreign key referencing AssemblyPlant(Code). ChassisNumber VARCHAR(6), -- Unique chassis number. FOREIGN KEY (CountryOfManufacture) REFERENCES AGENT_GATEWAY.AG_SCHEMA.CountryOfManufacture(Code), FOREIGN KEY (Manufacturer) REFERENCES AGENT_GATEWAY.AG_SCHEMA.Manufacturer(Code), FOREIGN KEY (VehicleBodyType) REFERENCES AGENT_GATEWAY.AG_SCHEMA.VehicleBodyType(Code), FOREIGN KEY (VehicleSeries) REFERENCES AGENT_GATEWAY.AG_SCHEMA.VehicleSeries(Code), FOREIGN KEY (YearOfManufacture) REFERENCES AGENT_GATEWAY.AG_SCHEMA.YearOfManufacture(Code), FOREIGN KEY (AssemblyPlant) REFERENCES AGENT_GATEWAY.AG_SCHEMA.AssemblyPlant(Code) );
-- Insert a sample VIN record into the VW_Type25_VIN_Master table. -- This entry corresponds to a vehicle manufactured in Germany by Volkswagen, -- with specific attributes decoded from the VIN. INSERT INTO AGENT_GATEWAY.AG_SCHEMA.VW_Type25_VIN_Master ( VIN, CountryOfManufacture, Manufacturer, VehicleBodyType, VehicleSeries, YearOfManufacture, AssemblyPlant, ChassisNumber ) VALUES ( 'WV2ZZZ25ZEH0000', 'W', 'V', '2', '5', 'E', 'H', '0000' ); |
After setting up the data, we need to create a semantic model. For this purpose I have created the following semantic model, with example query using natural language. Upload this file as .yaml to the public schema, which we created at beginning of the blog post.
name: Volkswagen
tables: - name: chassis_codes description: Production date and chassis number for each Type 25 vehicle. base_table: database: agent_gateway schema: ag_schema table: type25chassiscodes primary_key: columns: [month, year, chassisnumber] dimensions: - name: month expr: month description: Month of production data_type: varchar
- name: year expr: year description: Year of production data_type: number
- name: chassis_number expr: chassisnumber description: Chassis number identifier data_type: varchar
- name: vin_master description: Master VIN table for decoding Type 25 vehicle identification numbers. base_table: database: agent_gateway schema: ag_schema table: vw_type25_vin_master primary_key: columns: [vin] dimensions: - name: vin expr: vin data_type: varchar - name: country_code expr: countryofmanufacture data_type: varchar - name: manufacturer_code expr: manufacturer data_type: varchar - name: body_type_code expr: vehiclebodytype data_type: varchar - name: series_code expr: vehicleseries data_type: varchar - name: model_year_code expr: yearofmanufacture data_type: varchar - name: plant_code expr: assemblyplant data_type: varchar - name: chassis_number expr: chassisnumber data_type: varchar
- name: country description: Country code reference table. base_table: database: agent_gateway schema: ag_schema table: countryofmanufacture primary_key: columns: [code] dimensions: - name: country_code expr: code data_type: varchar - name: country expr: country data_type: varchar
- name: manufacturer description: Manufacturer reference table. base_table: database: agent_gateway schema: ag_schema table: manufacturer primary_key: columns: [code] dimensions: - name: manufacturer_code expr: code data_type: varchar - name: name expr: name data_type: varchar
- name: vehicle_body_type description: Body type reference table. base_table: database: agent_gateway schema: ag_schema table: vehiclebodytype primary_key: columns: [code] dimensions: - name: body_type_code expr: code data_type: varchar - name: description expr: description data_type: varchar
- name: vehicle_series description: Vehicle series reference table. base_table: database: agent_gateway schema: ag_schema table: vehicleseries primary_key: columns: [code] dimensions: - name: series_code expr: code data_type: varchar - name: description expr: description data_type: varchar
- name: model_year description: Year code to calendar year mapping. base_table: database: agent_gateway schema: ag_schema table: yearofmanufacture primary_key: columns: [code] dimensions: - name: model_year_code expr: code data_type: varchar - name: year expr: year data_type: number
- name: assembly_plant description: Assembly plant code reference. base_table: database: agent_gateway schema: ag_schema table: assemblyplant primary_key: columns: [code] dimensions: - name: plant_code expr: code data_type: varchar - name: location expr: location data_type: varchar
relationships: - name: vin_to_country left_table: vin_master right_table: country relationship_columns: - left_column: country_code right_column: country_code join_type: left_outer relationship_type: many_to_one
- name: vin_to_manufacturer left_table: vin_master right_table: manufacturer relationship_columns: - left_column: manufacturer_code right_column: manufacturer_code join_type: left_outer relationship_type: many_to_one
- name: vin_to_body_type left_table: vin_master right_table: vehicle_body_type relationship_columns: - left_column: body_type_code right_column: body_type_code join_type: left_outer relationship_type: many_to_one
- name: vin_to_series left_table: vin_master right_table: vehicle_series relationship_columns: - left_column: series_code right_column: series_code join_type: left_outer relationship_type: many_to_one
- name: vin_to_model_year left_table: vin_master right_table: model_year relationship_columns: - left_column: model_year_code right_column: model_year_code join_type: left_outer relationship_type: many_to_one
- name: vin_to_plant left_table: vin_master right_table: assembly_plant relationship_columns: - left_column: plant_code right_column: plant_code join_type: left_outer relationship_type: many_to_one
verified_natural_language_queries: - name: lookup_vin_details description: Return full decoded information about a VIN. query: | SELECT vm.vin, c.country, m.name AS manufacturer, b.description AS body_type, s.description AS series, y.year AS model_year, a.location AS assembly_plant, vm.chassisnumber FROM ag_schema.vw_type25_vin_master vm LEFT JOIN ag_schema.countryofmanufacture c ON vm.countryofmanufacture = c.code LEFT JOIN ag_schema.manufacturer m ON vm.manufacturer = m.code LEFT JOIN ag_schema.vehiclebodytype b ON vm.vehiclebodytype = b.code LEFT JOIN ag_schema.vehicleseries s ON vm.vehicleseries = s.code LEFT JOIN ag_schema.yearofmanufacture y ON vm.yearofmanufacture = y.code LEFT JOIN ag_schema.assemblyplant a ON vm.assemblyplant = a.code WHERE vm.vin = ''; parameters: - name: vin_code type: string description: VIN code to decode |
Now that we have two tools, let's do a test run of the actual Agent Gateway.
Agent Gateway Installation and configuration
Installation
This installation process follow instructions found from Github.
(agent) mheino@RWin-PF4J1VD3:~/aiagent$ python --version Python 3.10.12
pip install orchestration-framework |
The actual code is shown below, where we set up the Snowflake connection and create the tools. Use the values for Cortex Search and Cortex Analyst from the code examples provided above. Note that the better the tool descriptions are, the easier it is for the task planner to select the correct tool.
The interactive shell part is my own addition, as I'm running this from the terminal.
from agent_gateway import Agent from agent_gateway.tools import CortexSearchTool, CortexAnalystTool, PythonTool from snowflake.snowpark import Session import os from dotenv import load_dotenv
load_dotenv()
# Set environment variables os.environ["SNOWFLAKE_ACCOUNT"] = "Your Snowflake-account" os.environ["SNOWFLAKE_USERNAME"] = "agent_user" os.environ["SNOWFLAKE_PASSWORD"] = "agent_user" os.environ["SNOWFLAKE_DATABASE"] = "agent_gateway" os.environ["SNOWFLAKE_SCHEMA"] = "ag_schema" os.environ["SNOWFLAKE_ROLE"] = "cortex_user_role" os.environ["SNOWFLAKE_WAREHOUSE"] = "agent_wh"
# Retrieve environment variables and define connection parameters connection_parameters = { "account": os.getenv("SNOWFLAKE_ACCOUNT"), "user": os.getenv("SNOWFLAKE_USERNAME"), "password": os.getenv("SNOWFLAKE_PASSWORD"), "role": os.getenv("SNOWFLAKE_ROLE"), "warehouse": os.getenv("SNOWFLAKE_WAREHOUSE"), "database": os.getenv("SNOWFLAKE_DATABASE"), "schema": os.getenv("SNOWFLAKE_SCHEMA"), }
# Create Snowpark session snowpark = Session.builder.configs(connection_parameters).create()
# Define configurations for existing tools search_config = { "service_name": "VW_DOCUMENTS", "service_topic": "Volkswagen T2.5/T3 repair documents", "data_description": "Volkswagen T2.5/T3 repair documents with parts needed to replace", "retrieval_columns": ["CHUNK", "RELATIVE_PATH"], "snowflake_connection": snowpark, "k": 10, }
analyst_config = { "semantic_model": "volkswagen.yaml", "stage": "PUBLIC", "service_topic": "Volkswagen VIN", "data_description": "Information on how to deconstruct a VIN to identify Volkswagen model", "snowflake_connection": snowpark }
# Initialize existing tools vw_man = CortexSearchTool(**search_config) vw_vin = CortexAnalystTool(**analyst_config)
# Update the agent's tools snowflake_tools = [vw_man, vw_vin] agent = Agent(snowflake_connection=snowpark, tools=snowflake_tools, max_retries=3)
# Interactive shell def interactive_shell(): print("Interactive Agent Shell. Type 'exit' or 'quit' to end the session.") while True: try: user_input = input("You: ") if user_input.lower() in ['exit', 'quit']: print("Exiting interactive session.") break response = agent(user_input) print(f"Agent: {response}") except KeyboardInterrupt: print("\nExiting interactive session.") break except Exception as e: print(f"An error occurred: {e}")
if __name__ == "__main__": interactive_shell() |
Testing the tools works as follows. I’ve highlighted my commands for clarity and separated the responses. In this mode, the GatewayLogger (set to INFO) displays all the tools being used. In this particular case, the logger showed that only Cortex Search was used — which isn’t an ideal example of agentic orchestration.
(agent) mheino@RWin-PF4J1VD3:~/aiagent$ python3 agent3.py 2025-04-05 18:43:45,256 - AgentGatewayLogger - INFO - Cortex Search Tool successfully initialized 2025-04-05 18:43:45,256 - AgentGatewayLogger - INFO - Cortex Analyst Tool successfully initialized 2025-04-05 18:43:45,256 - AgentGatewayLogger - INFO - Cortex gateway successfully initialized Interactive Agent Shell. Type 'exit' or 'quit' to end the session.
You: what parts do I need for clutch fix
2025-04-05 18:43:56,433 - AgentGatewayLogger - INFO - running vw_documents_cortexsearch task Agent: {'output': 'For a clutch fix on a Volkswagen T2.5/T3, you will need parts such as a standard 228mm clutch friction disc, clutch release bearing, and possibly a heavy-duty clutch cross shaft kit. Additionally, you may need tools like a clutch alignment tool and gearbox oil.', 'sources': [{'tool_type': 'cortex_search', 'tool_name': 'vw_documents_cortexsearch', 'metadata': [{'RELATIVE_PATH': 'type25.pdf'}, {'RELATIVE_PATH': '5-speed-manual-gearbox-094-vw-transporter-dec-1982.pdf'}, {'RELATIVE_PATH': 'Owners_ManualWestfaliaT3WM_01_80_EN.pdf'}, {'RELATIVE_PATH': 'Bentley_Manual_Compressed.pdf'}, {'RELATIVE_PATH': '1980_VW_Transporter_Owners_ManuaVWT25WM.pdf'}]}]}
|
Let’s add the part which most likely will not work all the time e.g. our Python tool. We will introduce a new tool that will scrape eBay, Autoteilmarkt and Autodoc for part prices.
from agent_gateway import Agent from agent_gateway.tools import CortexSearchTool, CortexAnalystTool, PythonTool from snowflake.snowpark import Session import os import re import statistics import requests from dotenv import load_dotenv from bs4 import BeautifulSoup
load_dotenv()
# Set environment variables os.environ["SNOWFLAKE_ACCOUNT"] = "zueemdq-container-services" os.environ["SNOWFLAKE_USERNAME"] = "agent_user" os.environ["SNOWFLAKE_PASSWORD"] = "agent_user" os.environ["SNOWFLAKE_DATABASE"] = "agent_gateway" os.environ["SNOWFLAKE_SCHEMA"] = "ag_schema" os.environ["SNOWFLAKE_ROLE"] = "cortex_user_role" os.environ["SNOWFLAKE_WAREHOUSE"] = "agent_wh"
# Retrieve environment variables and define connection parameters connection_parameters = { "account": os.getenv("SNOWFLAKE_ACCOUNT"), "user": os.getenv("SNOWFLAKE_USERNAME"), "password": os.getenv("SNOWFLAKE_PASSWORD"), "role": os.getenv("SNOWFLAKE_ROLE"), "warehouse": os.getenv("SNOWFLAKE_WAREHOUSE"), "database": os.getenv("SNOWFLAKE_DATABASE"), "schema": os.getenv("SNOWFLAKE_SCHEMA"), }
# Create Snowpark session snowpark = Session.builder.configs(connection_parameters).create()
# Define configurations for existing tools search_config = { "service_name": "VW_DOCUMENTS", "service_topic": "Volkswagen T2.5/T3 repair documents", "data_description": "Volkswagen T2.5/T3 repair documents with parts needed to replace", "retrieval_columns": ["CHUNK", "RELATIVE_PATH", "PAGE_NUMBER"], "snowflake_connection": snowpark, "k": 10, }
analyst_config = { "semantic_model": "volkswagen.yaml", "stage": "PUBLIC", "service_topic": "Deconstruct or identify Volkswagen using VIN", "data_description": "Information on how to deconstruct a VIN to identify Volkswagen model", "snowflake_connection": snowpark }
def get_average_part_price(oem_number): GBP_TO_EUR = 1.17 HEADERS = { "User-Agent": "Mozilla/5.0 (Windows NT 10.0; Win64; x64)" }
def normalize_oem(oem): return re.sub(r"[^a-zA-Z0-9]", "", oem).lower()
def extract_price(text): match = re.search(r"([0-9]+[\.,]?[0-9]*)", text.replace(',', '.')) return float(match.group(1)) if match else None
def scrape_ebay_parts(oem): url = f"https://www.ebay.com/sch/i.html?_nkw={oem}+vw&_sacat=60200" response = requests.get(url, headers=HEADERS) soup = BeautifulSoup(response.text, 'html.parser') items = soup.select(".s-item") return [("ebay", extract_price(item.select_one(".s-item__price").text)) for item in items if item.select_one(".s-item__title") and item.select_one(".s-item__price") and oem.lower() in item.select_one(".s-item__title").text.lower()]
def scrape_autoteilemarkt_parts(oem): url = f"https://www.autoteile-markt.de/ersatzteile-suche?search_term={oem}" response = requests.get(url, headers=HEADERS) soup = BeautifulSoup(response.text, 'html.parser') items = soup.select(".article-list__item") return [("autoteile", extract_price(item.select_one(".article-list__price").text)) for item in items if item.select_one(".article-list__name") and item.select_one(".article-list__price") and oem.lower() in item.select_one(".article-list__name").text.lower()]
def scrape_paruzzi_parts(oem): url = f"https://www.paruzzi.com/uk/volkswagen/?zoektrefwoord={oem}" response = requests.get(url, headers=HEADERS) soup = BeautifulSoup(response.text, 'html.parser') items = soup.select(".product") return [("paruzzi", extract_price(item.select_one(".product-price").text)) for item in items if item.select_one(".product-title") and item.select_one(".product-price") and oem.lower() in item.select_one(".product-title").text.lower()]
def scrape_autodoc_parts(oem): url = f"https://www.autodoc.fi/autonosat/oem/{oem.lower()}" response = requests.get(url, headers=HEADERS) soup = BeautifulSoup(response.text, 'html.parser') prices = [] for price_elem in soup.select('.product-list-item__price .price'): match = re.search(r"([0-9]+,[0-9]{2})", price_elem.get_text(strip=True)) if match: try: price_float = float(match.group(1).replace(',', '.')) prices.append(("autodoc", price_float)) except ValueError: continue return prices
oem_clean = normalize_oem(oem_number)
all_prices = ( scrape_ebay_parts(oem_clean) + scrape_autoteilemarkt_parts(oem_clean) + scrape_paruzzi_parts(oem_clean) + scrape_autodoc_parts(oem_clean) )
prices_by_vendor = {} all_eur_prices = []
for vendor, price in all_prices: if price is None: continue eur_price = round(price * GBP_TO_EUR, 2) if vendor == "ebay" else round(price, 2) prices_by_vendor.setdefault(vendor, []).append(eur_price) all_eur_prices.append(eur_price)
if not all_eur_prices: return { "oem": oem_number, "average_price": None, "currency": "EUR", "prices_by_vendor": {}, "note": "No valid prices found" }
return { "oem": oem_number, "prices_by_vendor": prices_by_vendor, "average_price": round(statistics.mean(all_eur_prices), 2), "currency": "EUR" }
python_scraper_config = { "tool_description": "takes OEM part as input and returns the price of part if found", "output_description": "price of a Volkswagen OEM part", "python_func": get_average_part_price }
web_crawler = PythonTool(**python_scraper_config)
# Initialize existing tools vw_man = CortexSearchTool(**search_config) vw_vin = CortexAnalystTool(**analyst_config) vw_prt = PythonTool(**python_scraper_config)
# Update the agent's tools snowflake_tools = [vw_man, vw_vin, vw_prt] agent = Agent(snowflake_connection=snowpark, tools=snowflake_tools, max_retries=3)
def interactive_shell(): print("Interactive Agent Shell. Type 'exit' or 'quit' to end the session.") while True: try: user_input = input("You: ") if user_input.lower() in ['exit', 'quit']: print("Exiting interactive session.") break
response = agent(user_input)
# Check if the response is a dictionary if isinstance(response, dict): output = response.get("output") sources = response.get("sources")
if output: print("\n🛠️ Suggested Repair Parts:\n") print(output)
if sources: print("\n📚 Sources:") for source in sources: tool_name = source.get("tool_name", "Unknown Tool") print(f"- Tool: {tool_name}") metadata = source.get("metadata", []) for meta in metadata: if isinstance(meta, dict): page = meta.get("PAGE_NUMBER", "N/A") path = meta.get("RELATIVE_PATH", "N/A") print(f" • Page {page} – {path}")
else: # Fallback if agent returns string or another type print(f"\nAgent: {response}")
except KeyboardInterrupt: print("\nExiting interactive session.") break except Exception as e: print(f"⚠️ An error occurred: {e}")
if __name__ == "__main__": |
Testing the tool. This video is not sped up — you can see the Gateway takes its time to think and ultimately doesn’t understand my question about the Syncro (a different VW model).
Conclusion
This was a crude but functional demonstration of what’s possible with Snowflake’s Agent Gateway. We combined Cortex Search, Cortex Analyst, and a custom Python tool to build a multi-tool AI agent that can answer complex questions related to Volkswagen T25/T3 repair needs — based on real documents and live web data.
The biggest wins here come from having good tools — Python being the standout in this case — and, as always, from solid data quality. Without clean and structured data, even the smartest orchestration won’t give you great results.
While this was run from a terminal for simplicity, in a real-world use case we would’ve wrapped the whole thing with Streamlit to make it user-friendly. And since we’re already in Snowflake, we could’ve used Snowflake Container Services to host a production-ready version of the agent, as suggested in the official GitHub repo.
There are still a few limitations — like how Cortex Parse doesn’t chunk text, and how scanned PDFs can be messy — but the overall architecture is solid and extendable. With the right tools and the right data, this setup becomes a very capable assistant for diagnostics, VIN decoding, and spare part estimation.
|
|