Share to gain more social capita
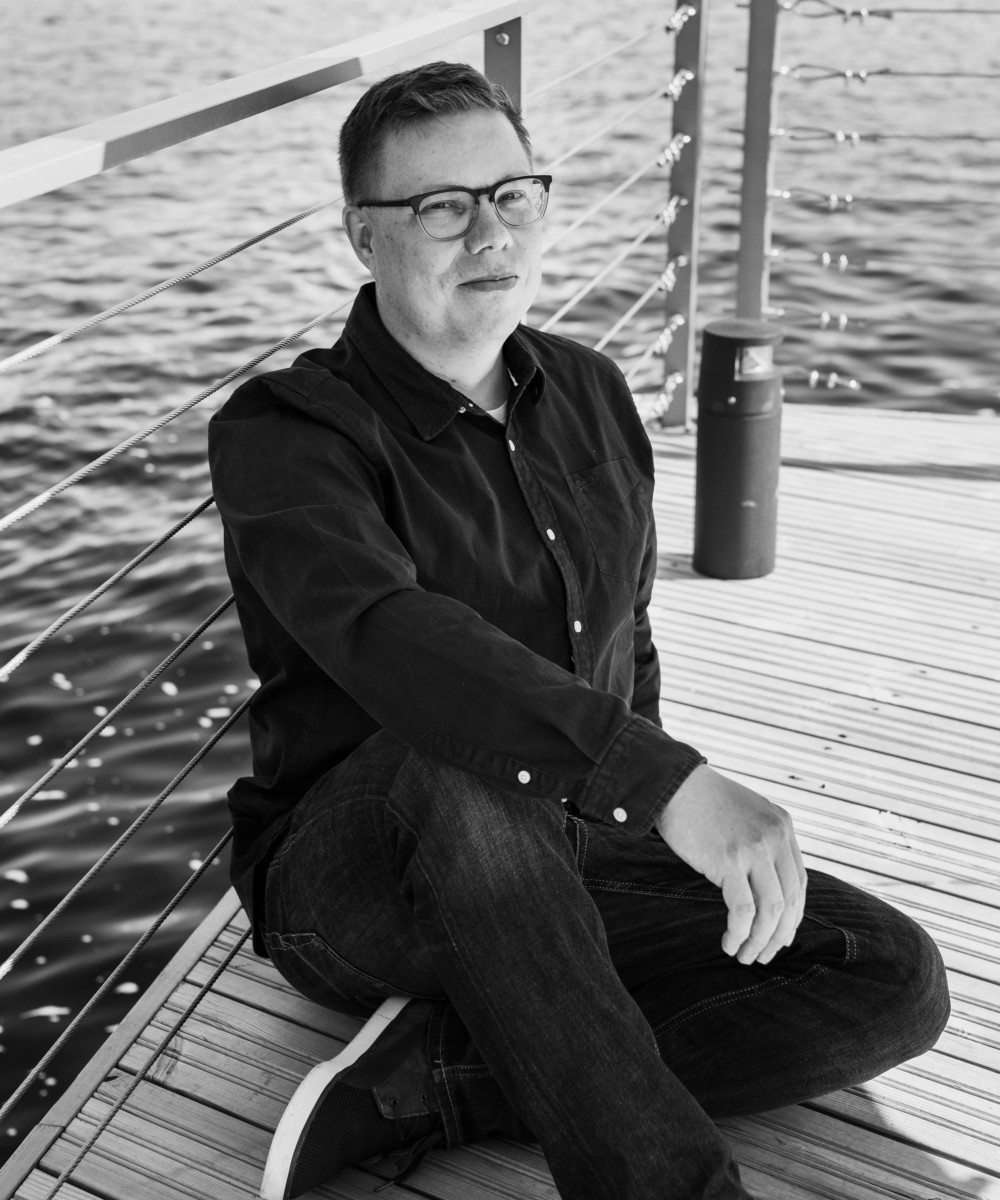
Written by — Kimmo Kantojärvi, Data Architect Partner
Energy optimization is a hot topic at the moment. With all the turbulence around business and higher inflation rates, I thought to discuss our view on energy optimization based on the customer cases we have going on.
Written by — Kimmo Kantojärvi, Data Architect Partner
Share to gain more social capita
For a long time, energy optimization has been 2-folded: either optimization of single machines/components (higher efficiency for example) or local, mostly manual optimization of a factory or similar-sized concept. This has been the case mainly because of the complexity of optimization of whole production lines consisting of multiple machines and the lack of proper tools. On the other hand, this setup has been “enough.” But things have changed due to increasing energy prices and limited energy supply. In addition, companies are looking for more sustainable ways of working and producing goods.
Below is an example optimization model of an extensive battery system. The picture is a simplified model, and typical energy optimization models are more complex, but the principles remain the same. First, you need some input data and a trained prediction model. With the input data, new predictions are calculated and based on these predictions, optimization is done. In the end, humans still typically have control of the final actions to be implemented.
To put it simply, the elements of the working model are:
Source: “Machine Learning Based Optimization Model for Energy Management of Energy Storage System for Large Industrial Park” https://www.mdpi.com/2227-9717/9/5/825/pdf”
A typical optimization model comprises of following data sets:
Historical production data is used to train the model to learn how different components consume energy and the correlations between different machines for a specific production setup. It is also essential to understand what has been produced at a particular point in time. Weather data is not needed in most use cases, but there are cases where for example, wind speed or outside temperature can affect the energy consumption of the production.
In order to forecast and optimize future energy consumption, production planning data is needed. It is important to know what kind of products are expected from the production lines so that the usage of different components in the production lines can be optimized. Changing the production planning based on energy optimization forecasts might also be needed.
Lastly, energy price data is needed to optimize the total cost of production. There are more and more service providers that are able to provide in addition to spot and intraday data longer-term price forecasts. One might need, for example, forecasts for gas, oil, weather forecast, or electricity prices.
From a technical point of view, the rapid development of new SaaS services and all kinds of machine learning libraries have opened up a whole new game for energy optimization. Whereas in the past, one would have to install complex software locally in the factory, data can now be connected from factories to cloud services and used for energy optimization in the cloud. Results can be returned to the factory automation systems to utilize the results in the production processes. There need to be security measures in place when connecting results back to the factory so that incorrect data does not end up screwing up the processes. This can be managed both automatically with data quality tools and by humans to control the processes. A more straightforward step is to provide the data as a reference to the process operators, who will then make the final decisions on how the process is run.
Cloud services allow scaling of the system for much more detailed data and higher frequency. For example, optimization runs can be run more often, thus providing better accuracy for optimization results. Also, connecting third-party data via cloud services is much more straightforward than it used to be. Getting accurate price forecasts with high frequency from external partners into your data platform is easy nowadays.
Currently, the most significant challenges are related to the premature nature of many SaaS services related to energy optimization. Because there is enormous interest and a lot of VC money available, many companies are hastily putting something together and selling the services while still in the beta phase. Therefore it is critical to select the right tools to ensure that your optimization system will be robust enough.
In addition, due to the nature of the energy optimization models, the connections to industrial processes required quite a work effort. Typically the connections to source data are behind many firewalls, and data standards are not that common. There are, of course, industry standards such as SCADA, OPC UA, and many others, but still, there are a lot of legacy systems in use. So building solid data pipelines from and back to the processes takes some time.
One of the projects we've been working on around energy optimization has been with UPM Energy. The former project lead at the energy services, Jukka-Pekka Häkli, describes the experiences:
The solution we've built together combines data from various internal and external sources, forecasts energy consumption, and trades energy on the markets. The platform has been a great success, and our customers are happy with the concrete results it provides.
We at Recordly want to extract value from your data and have a cost-efficient setup to optimize your energy consumption.